Overview: using PSVR on the PlayStation 5
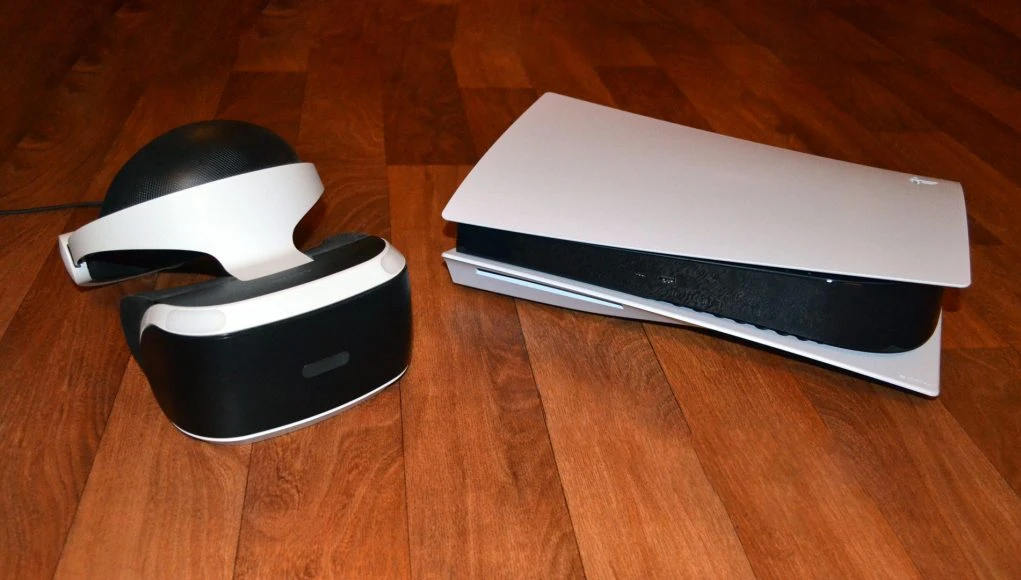
Overview: using PSVR on the PlayStation 5
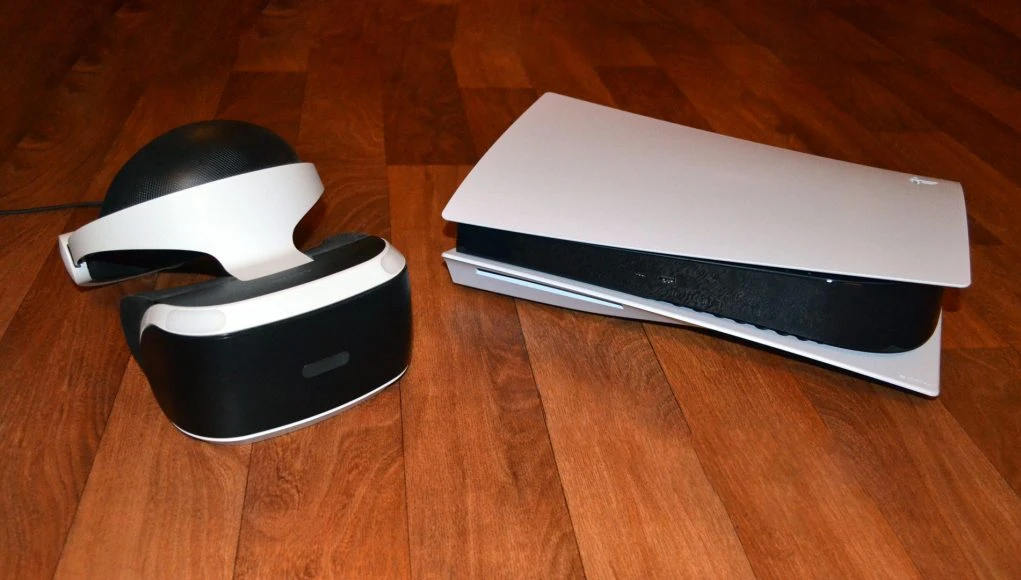
Since before the release of PS5 there are only a few days, many users are probably wondering how PSVR will work on the new console.
PS5 will provide full support PSVR due to backward compatibility with existing games PS4, created for the headset. Although you will not see big changes in PSVR immediately, load time - a definite improvement. Large changes can occur in the game through patches after release; and there is still great potential for native games for the PS5, created for PSVR, but so far Sony has not yet confirmed whether to allow developers to do this.
Setting PSVR on PS5
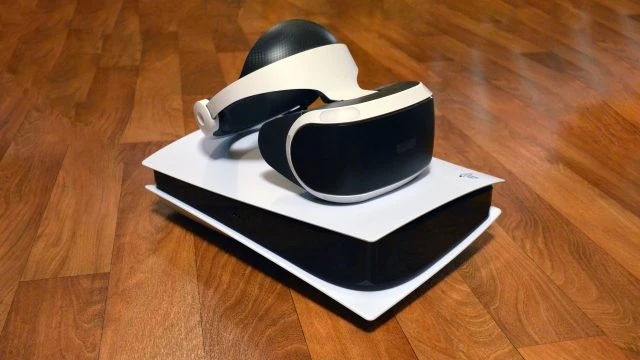
Requires camera adaptor
Setting PSVR in PS5 is the same process, which are all used on the PS4 (all the same connectors in all the same places), but with one key difference: you have to have the camera adaptor for PS5 PS4. Without it you will not be able to connect the camera PS4 to PS5, which is required for all AR games on the console. And unfortunately, according to Sony, the new camera PS5 will not work with the PSVR.
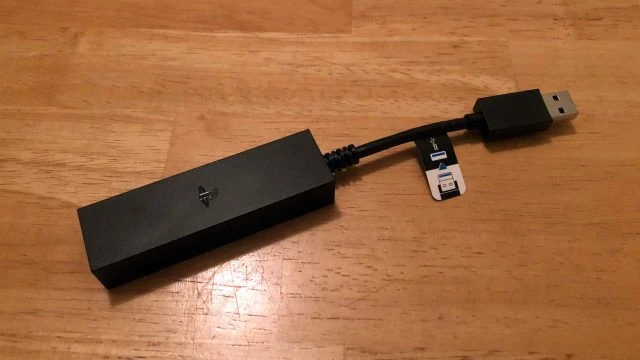
Sony provides a camera adapter PS5 PS4 for free; click on this page to make him a request.
Supported controllers
To use PS5 on PSVR you will also need a supported controller, which is not included controller to PS5. Supports the following controllers:
- PS4 controller DualShock
- PS Move
- PS Aim
It is a pity that the PS5 controller DualSense is not supported, because it is really impressive tactile feedback that'd look great in combination with the PSVR.
Setting
After you connect the PSVR to PS5 and turn it on, you will be greeted by the familiar setup screen where the new user will be able to properly install a headset and placed in the camera field of view. When you first connect the controller moving or aiming, you will be asked to hold it in front of the camera for calibration of the sphere tracking.
Installing games
Installing games on PS5 PSVR generally quite simple. After logging in to the console with an account PlayStation Network you will be able to see your digital PS4 games to their library of games and pick which ones you want to install.
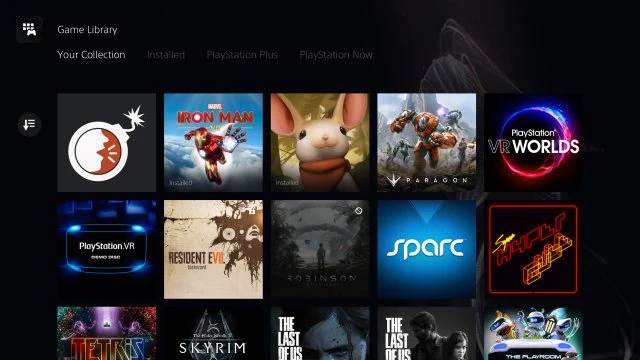
The library will show which of your games for PS4 compatible with your PS5; if the game is not compatible, you will see the symbol "stop" (🚫).
If you have a game for PSVR on the disk, it will be the same experience on PS4: insert the disk to install the game before playing.
No new options or features
PSVR for PS5 does not receive any new features or options, but you can expect the same settings that were used on the PS4, including the software IPD setting (and the ability to measure your IPD with the camera), the ability to see the control panel PS5 and play games that are not related to virtual reality, in the mode of "cinema", and also record videos and screen shots of virtual reality.
PSVR play on PS5
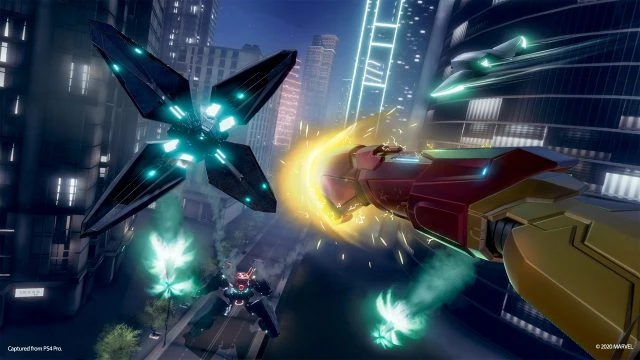
Play PSVR on PS5 is almost the same as playing on PS4 PSVR Pro; any improvements Pro used PS4 games on the PS5 VR (usually settings to improve the sharpness of the image).
Those who have tested a number of games PSVR, including those that use DualShock, Move and Aim, found no problems with the gameplay, and are unable to find any obvious graphical improvements compared to PS4 Pro. On the headset tracking is no different (which is not surprising, given that it uses the same controllers and camera).
However, there is one expected, but a valuable improvement: loading speed.
Yes, this fast SSD in PS5, which many have heard, greatly affects the amount of time that you spend looking at a loading screen in my headset. As far as improved boot time, depends on the game, but here are a few examples of tests:
The game - loading Screen | PS4 Pro | PS5 | Improvement |
Iron Man VR - the new game | 0:42 | 0:21 | 2x |
Iron Man VR-cinematic movie | 0:56 | 0:22 | 2.5 times |
Farpoint - Level Arrival | 0:35 | 0:18 | 1.9 times |
Farpoint - Intro | 0:13 | 0:06 | 2.2 times |
These figures coincide with the statements of Sony that PS5 of the memory bandwidth is 2.5 times higher than that of PS4 Pro.
Improved load times, of course, will be more important for games such as, for example, Iron Man VR. Reducing boot time in half or more do is of great importance for the pace of the game.
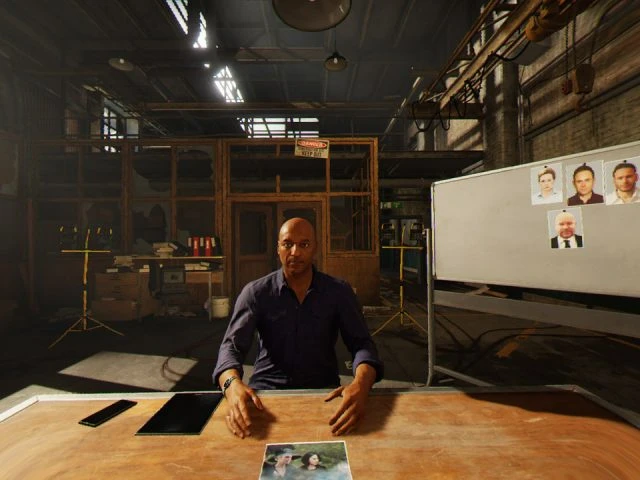
And although the company advertises even more performance gains in other areas PS5 that can significantly improve PSVR games on PS5, users will have to wait to see, reveal whether any updates after you run the potential backward compatibility of games PSVR on the console, not to mention whether we will get ever any games PS5 for PSVR.
Capture video and screen shots
And PS4 you can shoot video and capture screen shots PSVR in PS5 using the "Share" button on any controller you are using. Unfortunately, even on the PS5 they are removed from the same low resolution: 1280×720 for video and 960 x 720 for screenshots.
Source