Fan "of Mandalore" did not like the face of Skywalker and he fixed it better than the specialists at LucasFilm
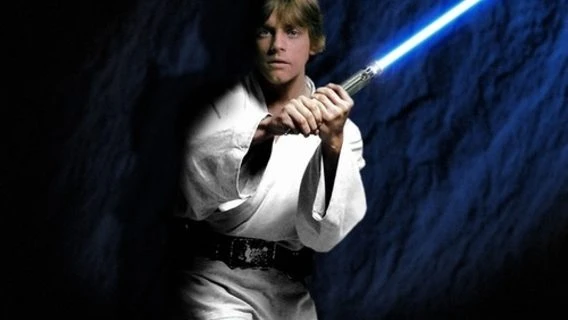
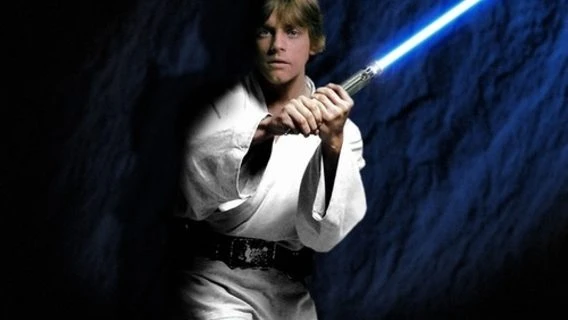
Disney+ released the last episode of the second season of "Mandalore" in which there was a Luke Skywalker - with the help of technology deepfake. Fan the picture quality is not liked, and he decided to improve it.
Fans criticized the Studio for what looked like a young Jedi. Then the author's YouTube channel Shamook has published a video in which he replaced the face of the hero with algorithms Deepfake. The result was better than it could make the employees of LucasFilm. Skywalker's face became more natural and his eyes were not as pale. According to Shamook, to improve the video it took just four days.