HOW TO ADD CUSTOM ENVIRONMENT IN OCULUS HOME OCULUS QUEST
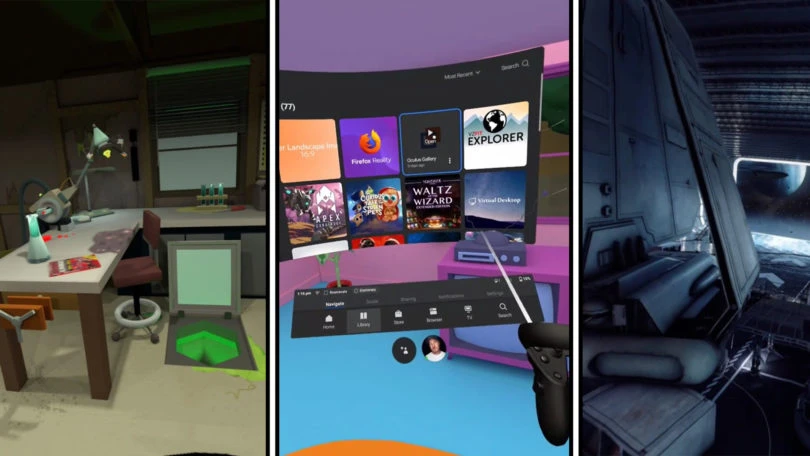
Thanks to the hard work of a few dedicated developers in the Oculus Quest, you can now experience the informal user environment Oculus Home.
Taking advantage of opportunities to side-loading Quest, you can now spice up your Oculus Home with a growing line of custom 3D environments taken directly from your favorite movies, TV shows and games. They include a living room from the Simpsons, a garage of Rick from Rick and Morty, the hangar of the Death Star from Star Wars, the flight deck of the ship N7 Normandy from Mass Effect and the Holodeck from Star Trek. Some of these environments even include music and animation.
Interested in changing your experience Oculus Home? Here's how to start adding custom environment:
Before you can start downloading the new environment, you need to remove the current default environment. The best way to achieve this is to use the app SideQuest for PC / Mac; just plug in your headset, refer to "Applications" and delete the com.oculus.environment.prod.rifthome ("Classic" Rift Home) or com.oculus.environment.prod.winterlodge.
From here, just download APK the environment you want to use and upload to a connected headset via SideQuest. If your environment is not loaded immediately, just select a different environment and then switch back.
Some of these custom environments even contain additional audio SFX and animation. For example, the Simpsons sing the theme song that runs on loop. Adding audio requires uploading of additional application settings, and then updates the virtual environment. Because third-party user environment for Quest are not officially supported Oculus, so we can expect some "glitches".
For a complete list of available environments and additional guides, check out the Oculus Discord Homes. Interested in the environment, but do not see it in the list? Read this guide on how to start creating your own 3D home environment.